Watch the video
AAPG ICE 2022: Structural Styles: Challenges in Seismic Imaging
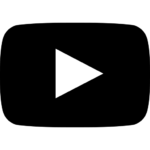
Vestrum, R.W., and Cameron, G.H., 2022, Structural Styles: Challenges in Seismic Imaging, AAPG ICE 2022, Cartagena, Colombia
This presentation develops the themes presented in Chapter 2 of the AAPG publication, Andean Structural Styles: A Seismic Atlas. Seismic data in areas like the Andes have unique challenges that break traditional seismic-imaging methods designed for offshore exploration. Reducing exploration risk in these basins requires a workflow tailored to the geologic setting. The under-constrained nature of the seismic data requires tight integration with the structural geologist.
Seismic imaging is a vital tool for mapping the complex geologic structures of the Andes. The method of imaging the Earth’s subsurface with seismic waves is powerful, and it has certain limitations—especially when deployed in complex-structure land areas like the mountain ranges and high plains of the Andes. Understanding the technologies involved and how they are applied to this specific geologic setting will improve our understanding of the risks and uncertainties involved in the interpretation of structures on seismic images.
Seismic data in thrust-belt environments are typically low data density and have low signal-to-noise ratios, all while attempting to image complex geologic structures. The data are acquired over rough topography with laterally varying velocities from the surface down. If the near surface is the lens through which we image the subsurface, our lens is bumpy and distorted. These are the challenges of seismic processing in fold thrust belts, and decades of technology development has gone into facing those challenges, from weathering corrections for the near-surface, to advance migration algorithms that can image below major thrust faults.